Scholars from the humanities and social sciences have been adopting digital methods into their respective fields. Large, publicly accessible datasets allow researchers to distill trends beyond individual occurrences of media phenomena. This use case provides researchers (and by extension investigative journalists) with practical methods to sift, connect and analyze various data and media collections in search of factual responses to broad societal research questions. Our aim is to provide AI-based tools that facilitate the identification of patterns or new research questions in aggregated, multi-modal collections. Based on the AI tools, we envision an infrastructure that will facilitate gathering and preparation of data to support macro-level analysis, e.g. the role of political and gender bias in media programs, and micro-level analysis, e.g. close reading of specific programs, bias effects of speaker selection, and topic presentation. This includes the use of (among others) Computer Vision analysis and Natural Language Processing, but goes beyond the detection of individual features, to equip researchers with reliable and explainable analyses and visualisations at aggregate levels.
This use case focuses on issues of framing, representation and bias: topics of great interest to social sciences and humanities. However, the definition and operationalization of such complex societal issues are itself subject to debate. Scholars will develop their own understanding and identify more specific instances of these broad phenomena, e.g. the degree to which talk show hosts interrupt female versus male speakers, or the representation of violence in news channels covering a conflict. To facilitate such research with AI-based tools requires a great degree of flexibility and configurability. At the core, the tools should enable researchers to perform basic operations: detect entities of various modalities within large multi-modal datasets. But for example, what exactly the tools detect, which confidence scores for results are to be used and in which combinations entities should occur should be configurable by the researchers.
Finally, academic research has the highest standards when it comes to transparency of methodologies, fairness of representation in training sets and research reproducibility. Therefore, this use case will also evaluate the pre-conditional requirements for trustworthy AI-based tooling, which allows scholars to confidently deploy the tooling.
Discover more on this topic on the whitepaper on AI Techniques and Tools for Social Sciences and Humanities Research.
Watch the Demo-Video
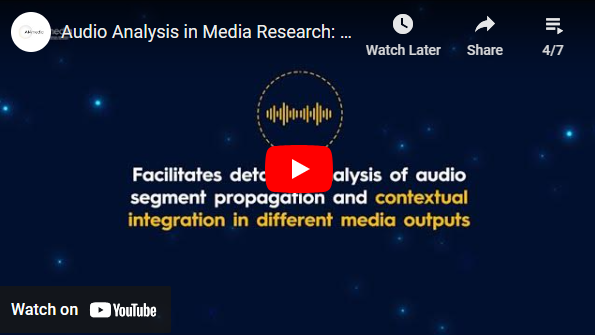